practice - torch sklearn numpy#
sklearn, numpy for linear regression and gradient descent
kaggle House Prices - Advanced Regression Techniques์์ ๊ฐ์ ธ์จ ๋ฐ์ดํฐ๋ฅผ ์ด์ฉํ์ฌ, Linear Regression์ ๊ตฌํํด๋ณด์.
์ฐ๋ฆฌ์ SalesPrice๊ฐ ๊ตฌํ๊ธฐ๋ฅผ ์ํ๋ y์ด๊ณ ์ด๊ฒ์ ์ฐ์์ ์ธ(continuous)ํ value์ด๊ธฐ ๋๋ฌธ์ linear regression์ ์ฌ์ฉํ๋ ๊ณผ์ ๋ผ๊ณ ๋ณผ ์ ์๋ค. GriLivArea(Above grade(ground) living area square feet)์ cs229์์ ๋งํ๋ size(feet^2)์ ๊ฐ์ฅ ์ ์ฌํ column์ด๋ผ๊ณ ์๊ฐ๋์ด์ ๋ฝ์๋ค. ๋จ์ํ๊ฒ scatter plot์ ํด๋ด๋ ์ฌ์ด๋๋ก ๋ง์ด ๋น ์ง ๋ช outlier๋ค์ ์ ์ธํ๋ฉด ์ด๋ ์ ๋์ linear ๊ด๊ณ๋ฅผ ๋ณผ ์ ์์ ๊ฑฐ๋ผ๊ณ ์๊ฐ๋๋ค.
import pandas as pd
import numpy as np
import matplotlib.pyplot as plt
from sklearn.linear_model import LinearRegression
from IPython.display import display, Markdown
train = pd.read_csv('./files/train.csv')
train
Id | MSSubClass | MSZoning | LotFrontage | LotArea | Street | Alley | LotShape | LandContour | Utilities | ... | PoolArea | PoolQC | Fence | MiscFeature | MiscVal | MoSold | YrSold | SaleType | SaleCondition | SalePrice | |
---|---|---|---|---|---|---|---|---|---|---|---|---|---|---|---|---|---|---|---|---|---|
0 | 1 | 60 | RL | 65.0 | 8450 | Pave | NaN | Reg | Lvl | AllPub | ... | 0 | NaN | NaN | NaN | 0 | 2 | 2008 | WD | Normal | 208500 |
1 | 2 | 20 | RL | 80.0 | 9600 | Pave | NaN | Reg | Lvl | AllPub | ... | 0 | NaN | NaN | NaN | 0 | 5 | 2007 | WD | Normal | 181500 |
2 | 3 | 60 | RL | 68.0 | 11250 | Pave | NaN | IR1 | Lvl | AllPub | ... | 0 | NaN | NaN | NaN | 0 | 9 | 2008 | WD | Normal | 223500 |
3 | 4 | 70 | RL | 60.0 | 9550 | Pave | NaN | IR1 | Lvl | AllPub | ... | 0 | NaN | NaN | NaN | 0 | 2 | 2006 | WD | Abnorml | 140000 |
4 | 5 | 60 | RL | 84.0 | 14260 | Pave | NaN | IR1 | Lvl | AllPub | ... | 0 | NaN | NaN | NaN | 0 | 12 | 2008 | WD | Normal | 250000 |
... | ... | ... | ... | ... | ... | ... | ... | ... | ... | ... | ... | ... | ... | ... | ... | ... | ... | ... | ... | ... | ... |
1455 | 1456 | 60 | RL | 62.0 | 7917 | Pave | NaN | Reg | Lvl | AllPub | ... | 0 | NaN | NaN | NaN | 0 | 8 | 2007 | WD | Normal | 175000 |
1456 | 1457 | 20 | RL | 85.0 | 13175 | Pave | NaN | Reg | Lvl | AllPub | ... | 0 | NaN | MnPrv | NaN | 0 | 2 | 2010 | WD | Normal | 210000 |
1457 | 1458 | 70 | RL | 66.0 | 9042 | Pave | NaN | Reg | Lvl | AllPub | ... | 0 | NaN | GdPrv | Shed | 2500 | 5 | 2010 | WD | Normal | 266500 |
1458 | 1459 | 20 | RL | 68.0 | 9717 | Pave | NaN | Reg | Lvl | AllPub | ... | 0 | NaN | NaN | NaN | 0 | 4 | 2010 | WD | Normal | 142125 |
1459 | 1460 | 20 | RL | 75.0 | 9937 | Pave | NaN | Reg | Lvl | AllPub | ... | 0 | NaN | NaN | NaN | 0 | 6 | 2008 | WD | Normal | 147500 |
1460 rows ร 81 columns
train[['SalePrice','GrLivArea']].plot.scatter(x='GrLivArea', y='SalePrice')
<Axes: xlabel='GrLivArea', ylabel='SalePrice'>
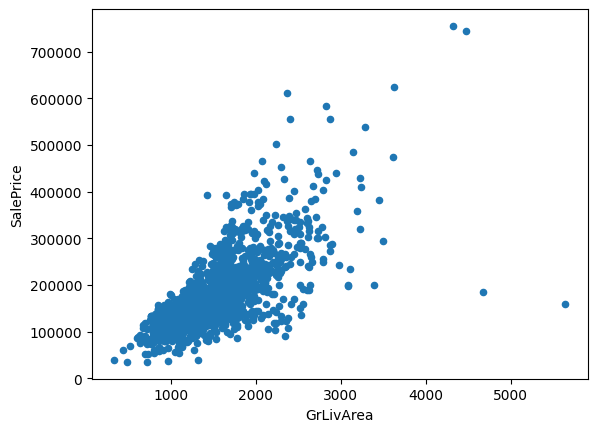
standardization#
\(\mu\)๋ ํ๊ท , \(\sigma\)๋ ํ์คํธ์ฐจ
\(z\)๋ ํ์คํ๋ ๊ฐ์ผ๋ก, ํ๊ท ์ผ๋ก๋ถํฐ ์ผ๋ง๋ ๋จ์ด์ ธ ์์ผ๋ฉฐ, ๊ทธ ๊ฑฐ๋ฆฌ๋ฅผ ํ์คํธ์ฐจ์ ๋ช ๋ฐฐ์๋งํผ ๋จ์ด์ ธ ์๋์ง๋ฅผ ๋ํ๋ธ๋ค.
๋ฐ์ดํฐ๋ฅผ ํ๊ท ์ด 0์ด๊ณ , ํ์คํธ์ฐจ๊ฐ 1์ธ ๊ฐ์ผ๋ก ๋ณํํ๋ ๊ฒ.
๋ฐ์ดํฐ์ ๋ฒ์๋ฅผ ์ผ์ ํ๊ฒ ์กฐ์ ํ๊ณ , ๋ค์ํ ์ค์ผ์ผ์ ๊ฐ์ง ๋ณ์๋ค๊ฐ ๋น๊ต ๊ฐ๋ฅํ๋๋ก ๋ง๋ฆ.
outlier์ ์ํฅ์ ๋ฐ์ ์ ์๊ธฐ ๋๋ฌธ์ ๋ฐ์ดํฐ์ ๋ถํฌ์ ๋ฐ๋ผ (๊ฐ์ฐ์์ normal distribution์ด ์๋ ๊ฒฝ์ฐ) ๋ค๋ฅธ scaler๋ฅผ ์ฌ์ฉํด์ผ ํ ์ ์๋ค.
์ ๋ ฅ๋ณ์ X๋ฅผ standardization ํ์ง ์๊ณ ํ์ตํ ๊ฒฝ์ฐ์ ๊ฐ์ค์น์ ๊ฐ์ด ์ ๋๋ก ํ์ต๋์ง ์์ ์ ์๋ค. ์ค์ผ์ผ์ด ๋ค๋ฅด๊ธฐ ๋๋ฌธ์.
X = train['GrLivArea'].values.reshape(-1,1)
y = train['SalePrice'].values.reshape(-1,1)
X = (X - X.mean()) / X.std()
y = (y - y.mean()) / y.std()
Sklearn linear regression#
lr = LinearRegression()
lr.fit(X, y)
y_pred = lr.predict(X)
plt.scatter(X, y)
plt.plot(X, y_pred, color='red')
plt.show()
# print("$h(\Theta)$" f"= {lr.coef_[0][0]:.2f}x + {lr.intercept_[0]:.2f}")
result_str = r"$h(\Theta)$ = {:.2f}x + {:.2f}".format(lr.coef_[0][0], lr.intercept_[0])
display(Markdown(result_str))
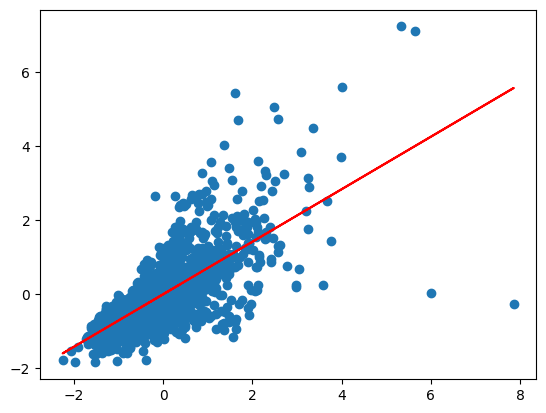
$h(\Theta)$ = 0.71x + 0.00
Numpy implementation#
lr = 1e-1
n_epochs = 5000
a = np.random.randn(1)
b = np.random.randn(1)
for epoch in range(n_epochs):
y_hat = a + b * X
error = y - y_hat
loss = (error**2).mean()
a_grad = -2 *error.mean()
b_grad = -2 * (X * error).mean()
a = a - lr * a_grad
b = b - lr * b_grad
result_str = r"$h(\Theta)$ = {:.2f}x + {:.2f}".format(b[0], a[0])
display(Markdown(result_str))
$h(\Theta)$ = 0.71x + 0.00
manim test#
# from manim import *
# from manim import config; config.media_embed=True
# %%manim -v WARNING --progress_bar None -r 400,200 --format=gif --disable_caching HelloManim
# class HelloManim(Scene):
# def construct(self):
# self.camera.background_color = "#ece6e2"
# banner_large = ManimBanner(dark_theme=False).scale(0.7)
# self.play(banner_large.create())
# self.play(banner_large.expand())